Security
Machine vs. machine
Greetings from Mav, trusted partner for your AI journey. This AI-generated moose has been on the hunt for threats and keeping security top of mind….
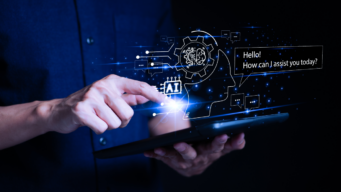
Navigating the Intersection of AI and Financial Risk: A Proactive Approach
Don’t miss out on the opportunity to transform your organization’s approach to data security, privacy, and governance in the age of GenAI.
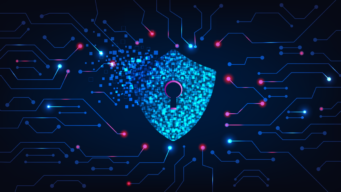
Cybersecurity Services combat an APT with NDR
Attackers linked to Iran and China are actively targeting critical infrastructure. Both the U.S. Environmental Protection Agency and National Security Agency have requested that each…
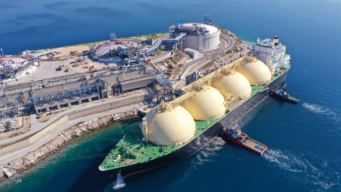
OpenText Summit | Houston Energy 2024 summary & key takeaways
A deep dive into the future of energy operations with AI
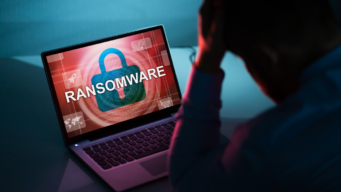
The Change Healthcare Cyberattack: A Wake-Up Call for Healthcare Cybersecurity
The attack on Change Healthcare is a pivotal moment for healthcare cybersecurity.
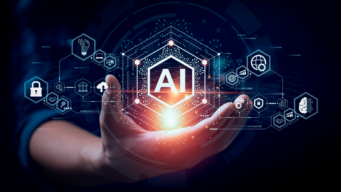
Increase speed and accuracy with AI driven static analysis auditing
We are thrilled to announce the next generation of Audit Assistant, our innovative machine-learning–assisted auditing of SAST results. Fortify™ now unlocks and reproduces contextual awareness…
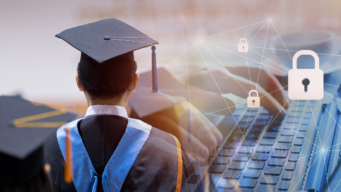
Strengthening Higher Education Institutions against evolving cyberthreats
As cyberthreats continue to evolve, it is crucial for higher education institutions and universities to be vigilant. Enforcing security strategies prudently designed to safeguard digital…
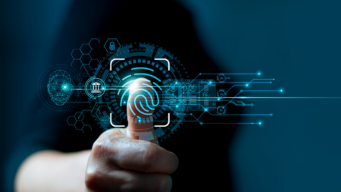
Streamline identity management with a secure automated cloud solution by NetIQ Identity Manager by OpenText
OpenText™ Cloud Editions (CE) 24.1 introduces exciting updates and enhancements tailored for CE customers. Among these notable additions is NetIQ™ Identity Manager, a cloud-based solution…
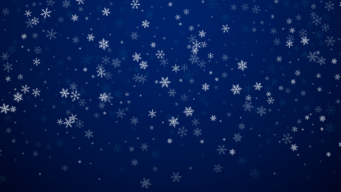
‘Twas the night before Christmas… An OpenText Voltage Poem
‘Twas the night before Christmas, when all through the house A hacker was stirring, and clicking his mouse; The stockings were hung by the chimney with…
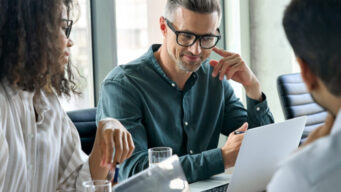
Strengthening cyber resilience
Cyberattacks are on track to cause $10.5 trillion a year in damage by 2025. That’s a 300 percent increase from 2015 levels. A robust cybersecurity…
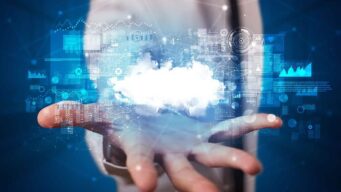
Meeting the new challenges of digital evidence management
Dealing with the scale and complexity of evidence in rich-media formats (images, video and audio) is one of the key challenges in evidence management for…
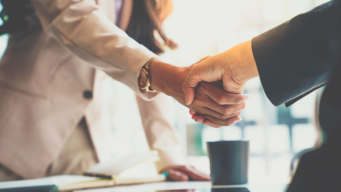
Fortify and Mobb join forces for faster fixes in SAST
As developers, we’re always striving to ship our code quickly while still maintaining the highest security standards. This balancing act can be tricky, as discovering…
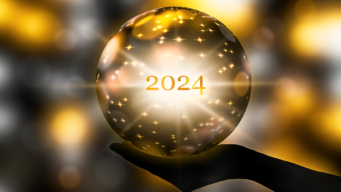
Predictions for the financial services industry in 2024
The word ‘rollercoaster’ best sums up 2023. We’ve experienced economic, geopolitical, technological, and societal challenges over recent months with the ongoing cost-of-living crisis, high interest…
Stay in the loop!
Get our most popular content delivered monthly to your inbox.